Speaker:- Prof. Dr. Jakob Runge
Head of Causal Inference group, Institute of Data Science, German Aerospace Center, Jena, Germany and Guest professor of Climate Informatics, Faculty of Electrical Engineering and Computer Science, Technische Universität Berlin, Germany
Title:- Causal inference for climate research
In the past decades machine learning has had a rapidly growing impact on many fields of natural-, life- and social sciences as well as engineering. Climate research is one of the more recent fields of application. Machine learning excels at classification and regression tasks from complex heterogeneous datasets and can answer questions like "What statistical associations or correlations can we see in the data?", "What objects are in this picture?", or "What is the most likely next data point?". But many questions in science, engineering, and politics, and especially in climate research, are about "What are the causal relations underlying the data?" or "What if a certain variable changes or is changed?" or "What would have happened if some variable had another value?". Data-driven machine learning alone fails to answer such questions. Causal inference provides the theory and methods to learn and utilize qualitative knowledge about causal relations. Together with machine learning it enables causal reasoning given complex data.
Furthermore, causal methods can be used to intercompare and validate physical simulation models. In this talk I will present an overview of this exciting and widely applicable framework and illustrate it with some examples from Earth sciences and beyond.
Short bio:
Jakob Runge heads the Causal Inference group at the German Aerospace Center’s Institute of Data Science in Jena since 2017 and is guest professor of computer science at TU Berlin since 2021. His group combines innovative data science methods from different fields (graphical models, causal inference, nonlinear dynamics, deep learning) and closely works with experts in the climate sciences
Meeting Recording:
Access Passcode: rcu&Tv87
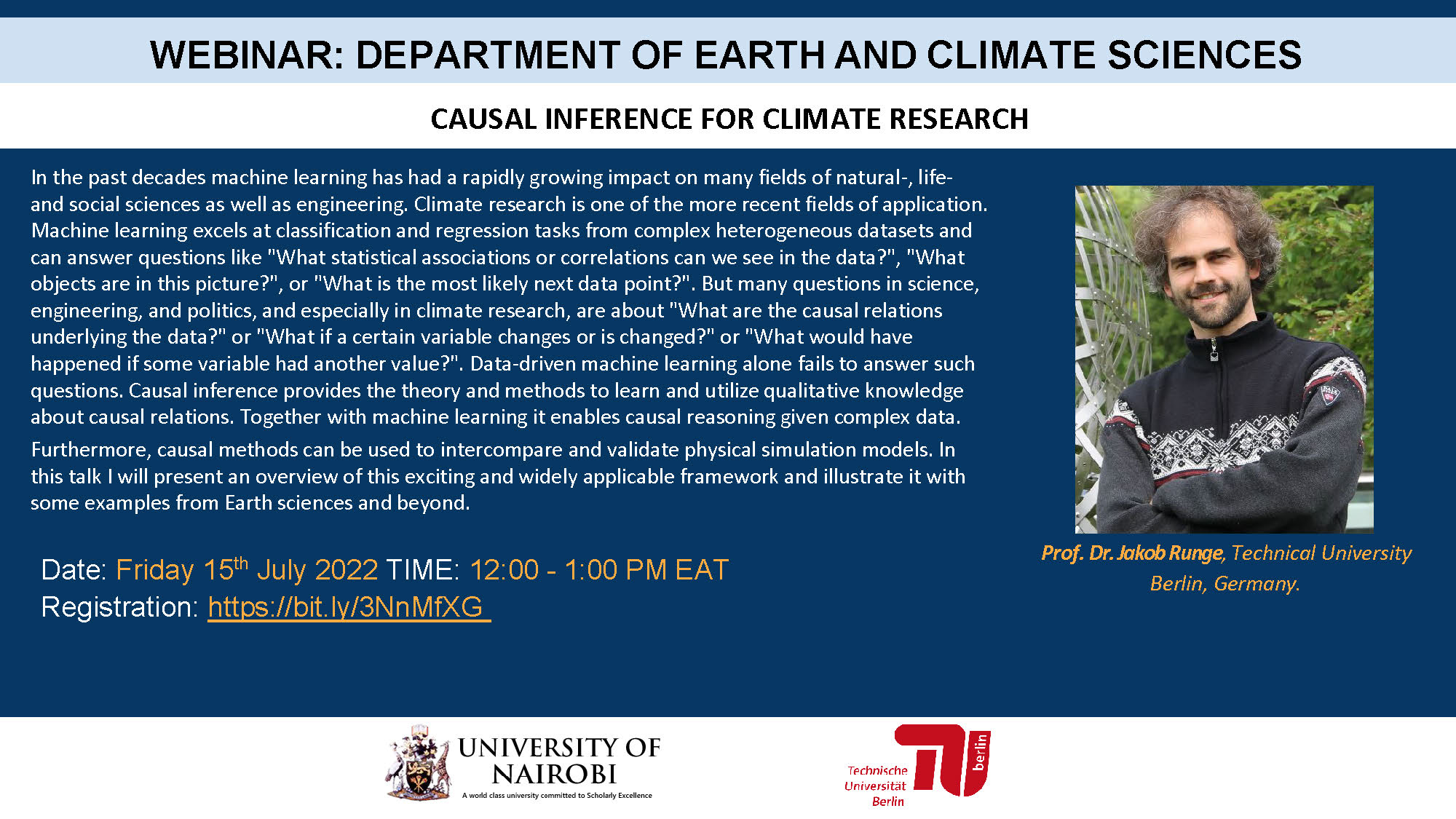